Copyright © 2024
Adaptive ML, Inc.
All rights reserved
Adaptive ML, Inc.
All rights reserved
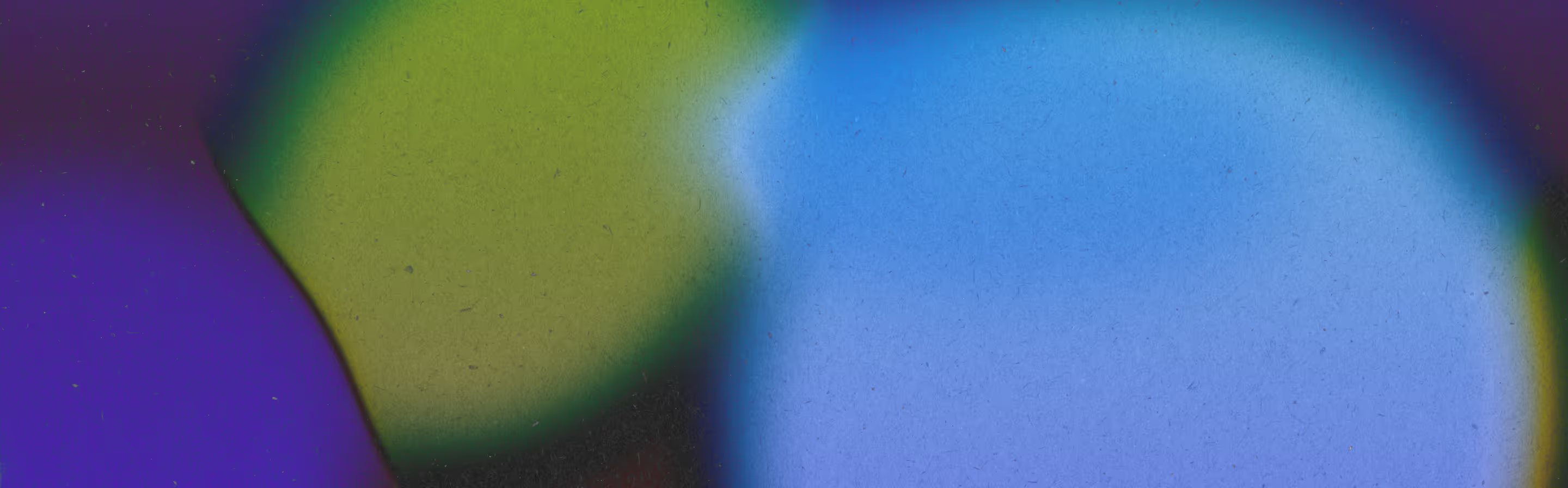
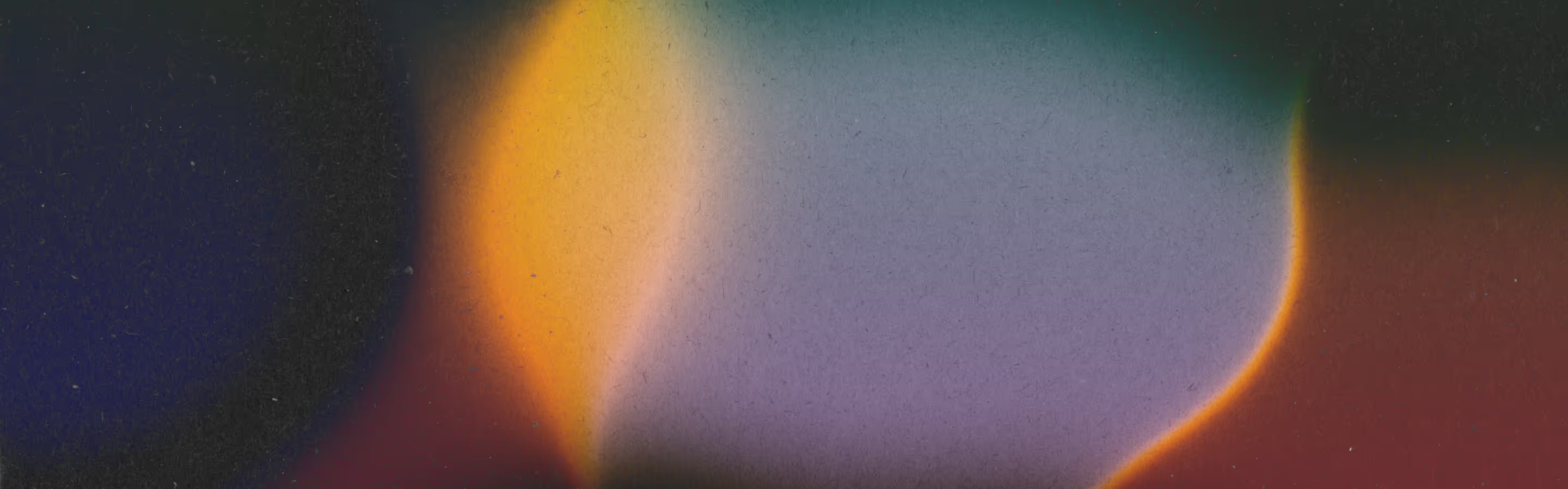

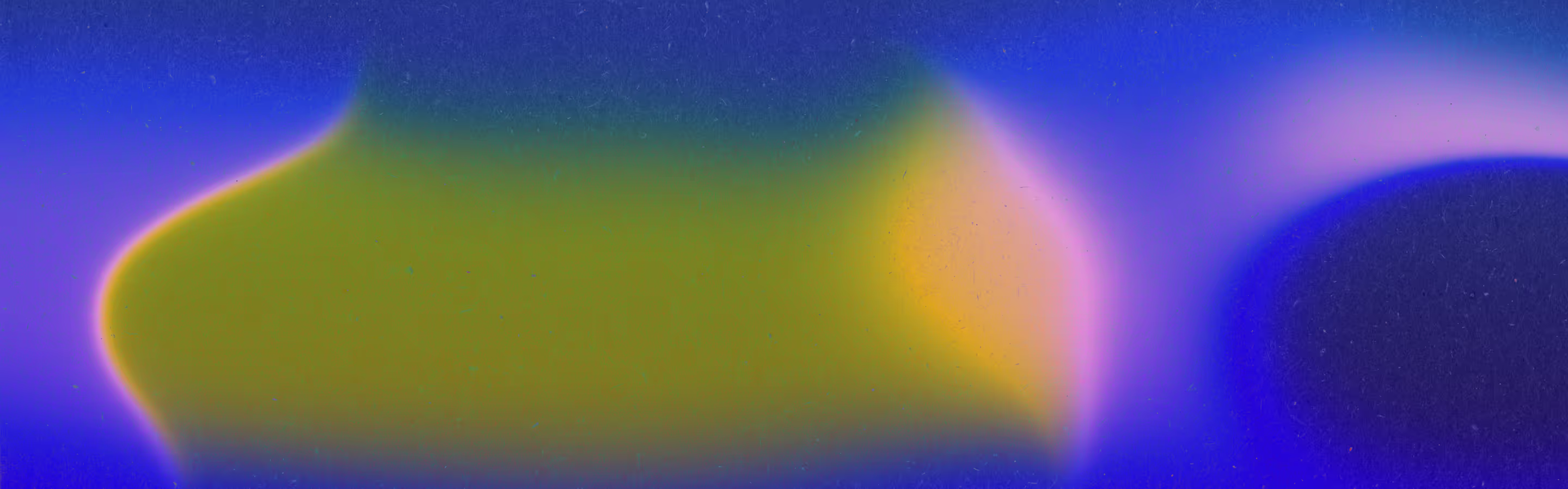
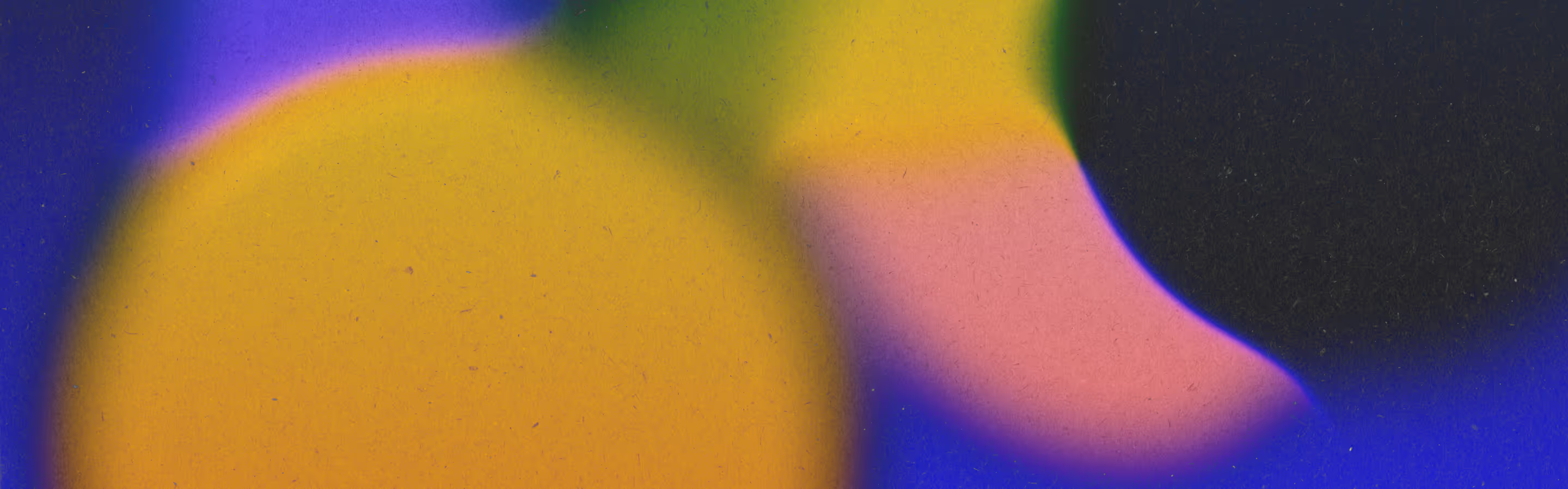
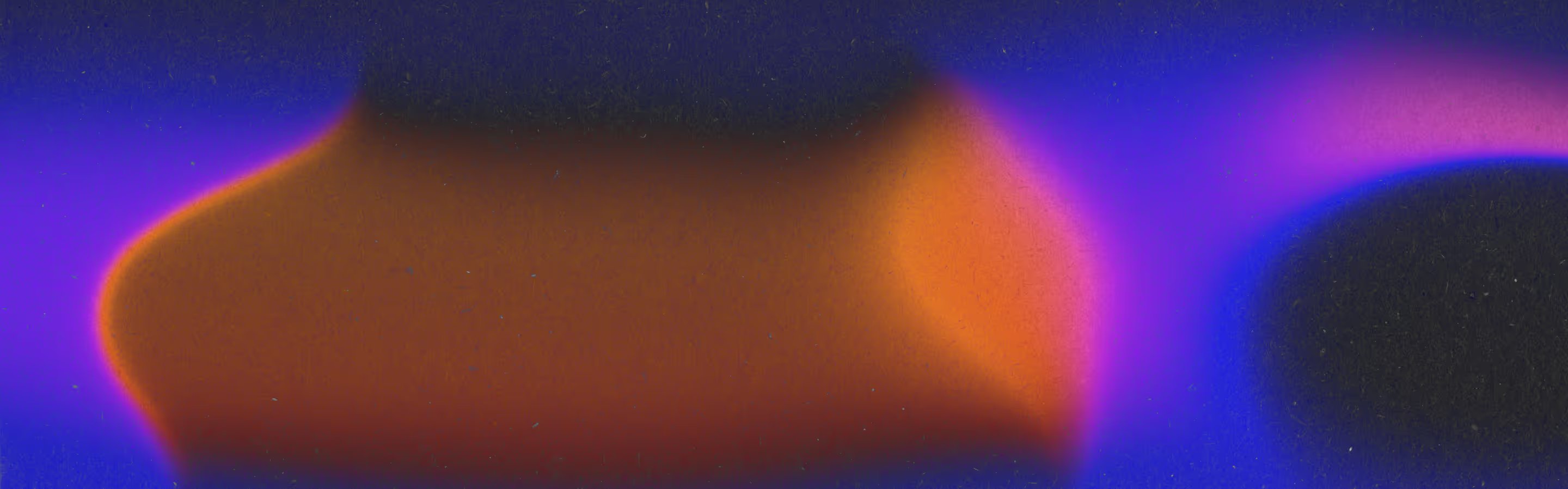
AT&T Selects Adaptive Engine to Build and Deploy Enterprise Reasoning Models
AT&T has engaged Adaptive ML, deploying Adaptive Engine as their reinforcement tuning platform for open-source models. Engineers and data scientists will use Adaptive Engine’s reinforcement learning capabilities to develop reasoning models tailored to their enterprise tools and knowledge.
AT&T is at the leading edge of developing and deploying GenAI in the telecommunications space, recently topping the BIRD-SQL benchmark for their work on text-to-SQL.They have deployed both proprietary APIs and open-source models to production across a variety of business units, driving significant gains in efficiency and execution accuracy.
As experienced GenAI users, AT&T recognizes that prompt engineering isn’t always enough to get to production; fine-tuning is required to deliver the precision, personalization, and performance necessary for production-grade LLMs. Fine-tuning also gives AT&T the ability to distill the capabilities of large models into smaller, cost-efficient ones, reducing GPU requirements and lowering costs without sacrificing quality.
AT&T has identified 50+ use cases where fine-tuning will be required, ranging from AskData (an internal text-to-SQL analysis application) to customer support, call summarization, document RAG, and more.
Their ultimate aim is to tune personalized reasoning models that are able to interact effectively with their business systems. To do so, they will leverage advanced reinforcement learning techniques in Adaptive Engine, such as PPO and GRPO, as well as test-time search, execution feedback, and synthetic data/environment generation.
They will also employ Adaptive Engine’s suite of evaluation tools, including bespoke AI judges and A/B testing, to ensure best-in-class model performance and safety.
For example, during AT&T’s evaluation period of Adaptive Engine, a Llama 3.1 8B was fine-tuned to improve factuality and helpfulness on RAG for telco documents. These highly-technical documents are critical for managing telco operations, but their complex format and use of industry-specific terminology makes it difficult for out-of-the-box LLMs to accurately retrieve and utilize information for RAG workflows.
The tuned model achieved a 51% win rate vs a leading closed-source LLM provider out of the box, meaning the fine-tuned model’s answer was more faithful and accurate to the RAG corpus than the LLM provider’s answer 51% of the time. Meanwhile, the LLM provider’s answer was preferable only 29% of the time, and the models tied in the other 20% of cases.
The ability to rapidly create, tune and deploy reasoning models tuned to the specificities of AT&T’s business will unlock unprecedented personalization and performance. Once deployed, these tuned models will also learn continuously from production feedback, such as user preferences and business metrics, further enhancing model accuracy and specificity.
Learn more about how AT&T is leveraging GenAI or book a demo of Adaptive Engine.